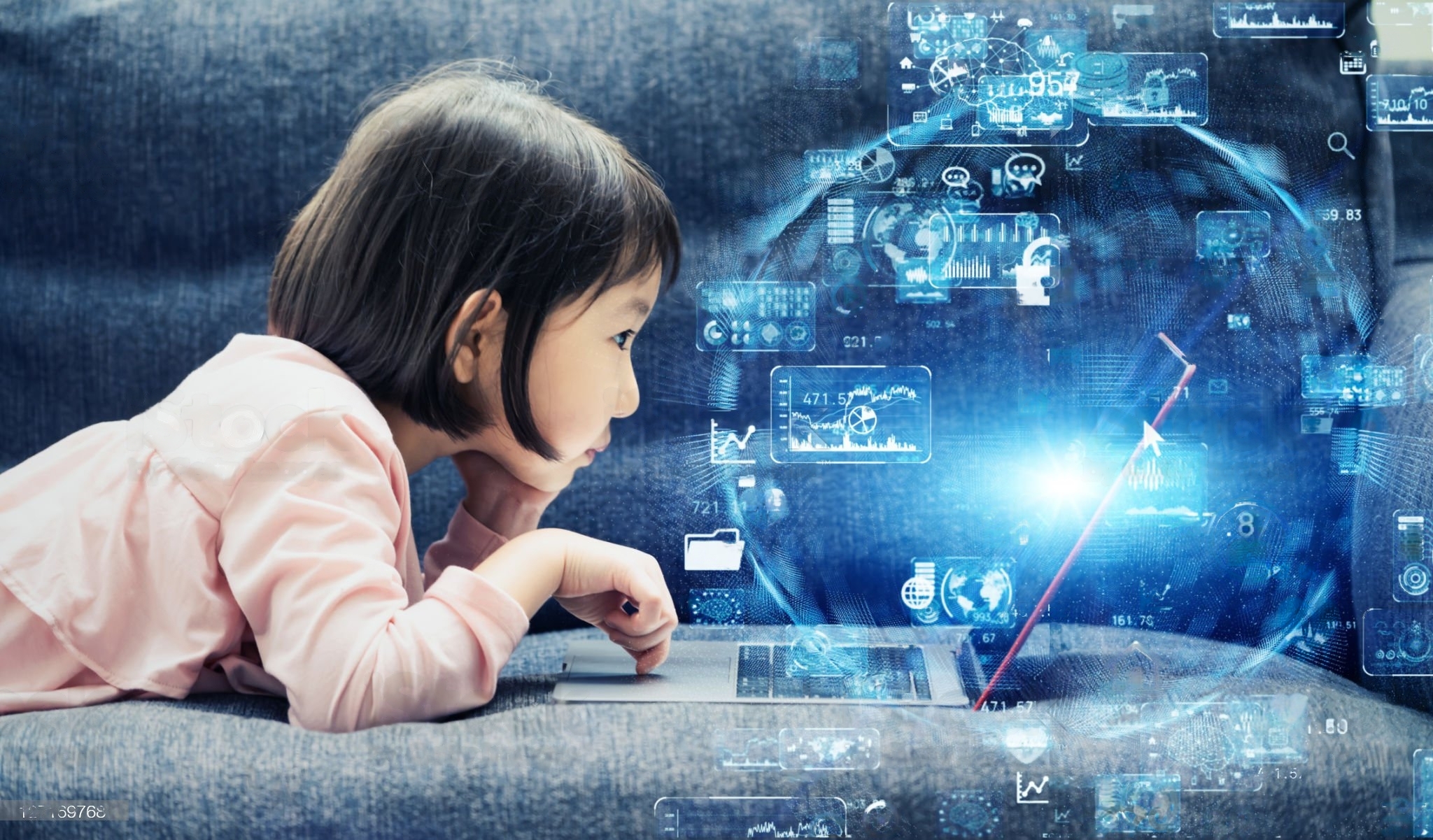
One Weird Trick to Accelerate AI Learning! The Meta-Learning Breakthrough
Artificial Intelligence (AI) has transformed the relationship between humans and technology, leading every industry in a new direction. However, one of the persistent challenges that AI poses for users is the time-consuming process of training AI models. It often requires massive amounts of data and computational power. It has led to another innovative approach, which is the learning-to-learn methodology. It can potentially revolutionize the learning speed of AI systems to acquire new knowledge and skills.
The Learning-to-learn methodology is often referred to as meta-learning. It enables models to learn new tasks with fewer examples and iterations. Rather than specializing in a single job, meta-learning equips AI with the ability to generalize across tasks, making it more adaptable and efficient. It has been inspired by human learning. Like humans, it also learns from past experiences and quickly grasps new concepts. By giving training on diverse tasks, AI models can develop their repository of knowledge that can help them accelerate their learning curve on various tasks. So let's deep dive into it.
What are the key mechanisms for faster learning?
1. Few-Shot Learning
Traditional AI models require huge data to achieve accuracy in the given task. However, meta-learning challenges it by training AI models on various smaller tasks. It helps them learn from a limited set of examples and grow their knowledge database. This ability is especially advantageous in situations where gathering substantial volumes of data poses difficulties.
2. Transfer and Generalization
Learning-to-learn methodology equips a system with more flexible and versatile learning capabilities, enabling it to transfer its acquired knowledge and skills to various domains. This leads to faster adaptation and reduced learning time when confronted with previously unseen tasks.
3. Model Architecture
Meta-learning isn't just about learning how to do specific tasks better; it's also about learning how to design better models. AI systems can learn which model architecture works best for different problems. This offers better responses in a lesser amount of time and cost for different tasks.
But what are its Challenges?
1. Task Selection
The success of meta-learning depends on the various tasks used for its training. The better the task will be given, the better its knowledge database will become.
2. Data Efficiency
While meta-learning reduces the need for huge data, it's still significant to ensure that the tasks and examples the developer chooses for its training are pertinent to real-world scenarios. Otherwise, their AI model will also give dreamy answers.
3. Hyperparameter Tuning
Meta-learning involves fine-tuning various hyperparameters that affect various factors, such as how long model architecture will take time to train, how much difficulty it will face to work along with its associated devices, or how well it generalizes to new data. Proper tuning leads to optimal model performance without overfitting or underfitting. Striking the right balance is crucial for optimal performance.
What are its prospects?
1. Personalized AI
Learning-to-learn can open doors for AI systems to rapidly adjust to the unique preferences and requirements of each user. It will enhance user experiences across various domains like Banking, Finance, Insurance, Power transmission, etc. Some examples of its usage in the power utility domain are - Energy Management and Forecasting, Demand Response programs, and Grid stability resilience.
2. Real-Time Adaptation
Their quick learning ability could facilitate real-time decision-making in dynamic environments, such as driverless cars swiftly responding to varying road conditions.
3. Resource Efficiency
The quick-learning AI models will reduce the demand for computational resources and energy consumption. This is one of the reasons that make AI more sustainable and accessible in environments with limited resources.
Conclusion
To sum up, the learning-to-learn methodology transforms AI through swift adaptation to novel tasks. This pivotal advancement has the potential to elevate AI beyond its current constraints, leading to industry transformations and even exceeding human learning capacities.